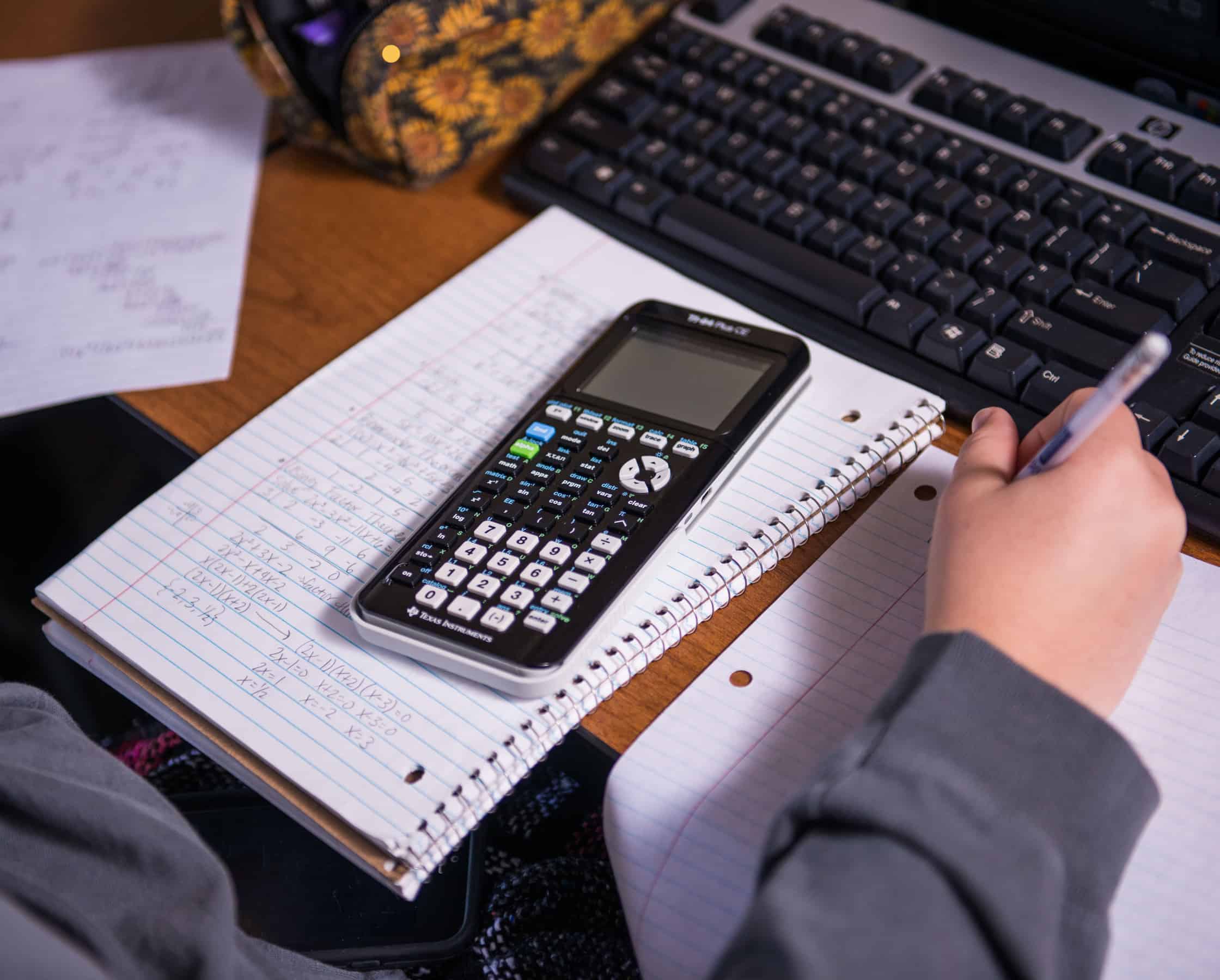
- On this page:
-
Researchers
-
Downloads
-
Results and Metrics
-
Publications
Repository of Image Databases with Augmented Features
Welcome to the Repository of Image Databases with Augmented Features. Here you can find ready to use with your classifier image databases with augmented image features. You may also augment the image features in your own image databases using the software tools found here.
Objectives:
- Software which allows users to augment the features of existing image databases to improve machine learning effectiveness and increase statistics.
- Links to download image databases with augmented features.
Contributors
This project is supported by the Department of Mathematics at East Texas A&M University. Please visit the department webpage for more information about Mathematics at East Texas A&M University.
Main Contributors
- Nikolay Metodiev Sirakov a
- Adam Bowden a
Collaborators
- Mengzhe Chen
- Oluwasey Ingbassani
- Long Ngo b
a. East Texas A&M University
b. L2TI, University Sorbonne Paris Nord, France
Downloads
- ELPAC-S
- SRWC (Sparce Representation Wavelet Classification) – Used in [8] for classification of augmented image databases.
- COIL100
- digit-MNIST [12] Training Images
- digit-MNIST Testing Images
- ISIC 2018 [10, 11]
- ISIC 2020 Training Images
- ISIC 2020 Testing Images
- COIL100
- digit-MNIST Training Images
- digit-MNIST Testing Images
- fashion-MNIST Training & Testing Images
- ISIC 2020 Training Images
- ISIC 2020 Testing Images
When using any results, software, or databases from this webpage, please cite the corresponding paper in which the results, software, or databases have been published (see reference section below), along with the following:
N.M. Sirakov, A. Bowden, T. Wang, J. Gamez, Repository of Image Databases with Augmented Features, https://www.tamuc.edu/projects/augmented-image-repository/?redirect=none
When using any databases linked here, cite the original databases [1, 5, 7] as well.
When using the ELPAC-S software, please include this citation:
A. Bowden, N.M. Sirakov, Active Contour Directed by the Poisson Gradient Vector Field and Edge Tracking, Journal of Mathematical Imaging and Vision – Springer, 63:665–680, 2021, https://rdcu.be/cflaI, (2021)
When using the SRWC software, include citation [8].
Sample Results and Metrics
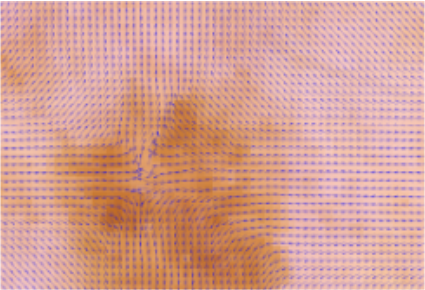
Below are some sample results from the related research work demonstrating the effectiveness of augmenting database images with more features. More information can be found in papers [6, 8, 9] listed in the reference section below. In particular, the following table contains results from our paper [6].
Model | ISIC 2020 [1] Original Dataset Accuracy (%) | ISIC 2020 vû Augmented [6] Accuracy (%) | Model | COIL-100 [7] Original Dataset Accuracy (%) | COIL-100 vû Augmented [6] Accuracy (%) | |
DenseNet 121 [2] | 89.40 | 88.88 | DenseNet 121 | 88.72 | 87.88 | |
ResNet 50 [3] | 90.20 | 91.19 | ResNet 50 | 89.51 | 90.16 | |
Inception ResNet V2 [4] | 89.60 | 92.50 | Inception ResNet V2 | 88.91 | 91.45 | |
EfficientNet B0 [5] | 91.69 | 92.90 | EfficientNet B0 | 90.99 | 91.85 | |
SeyNet [6] | 90.10 | 93.25 | SeyNet | 89.41 | 91.27 |
Sample COIL-100 Images (click for larger images)
Vector Field vû
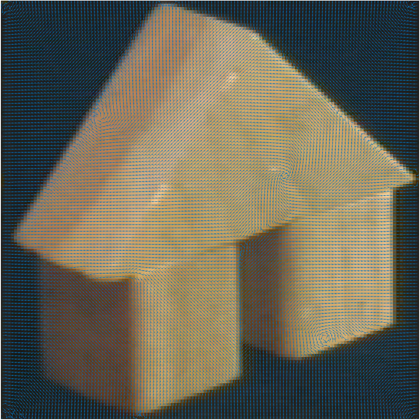
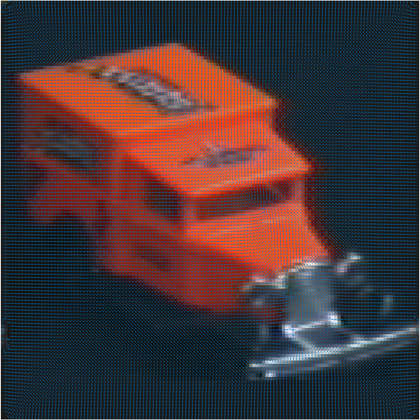
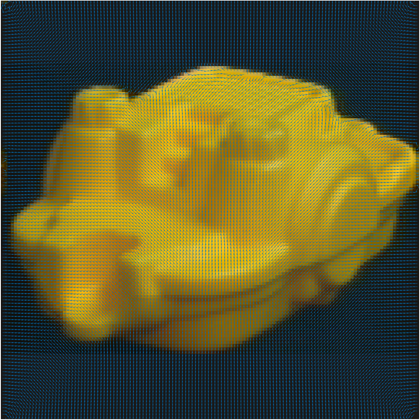
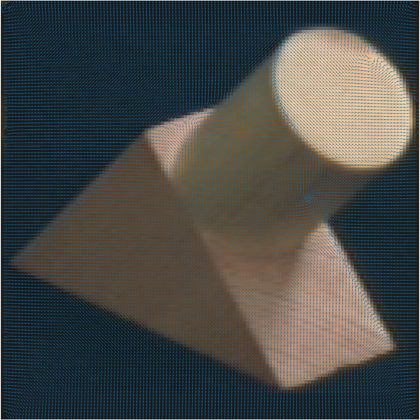
Vector Field vϕ̂
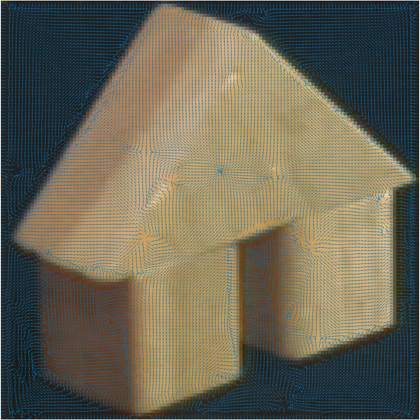
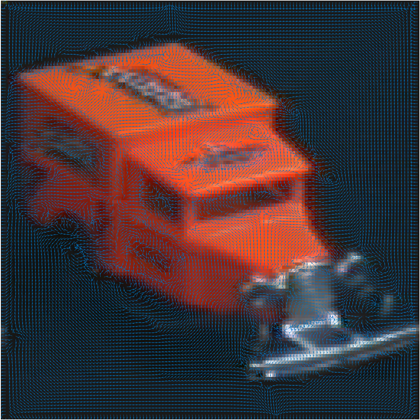
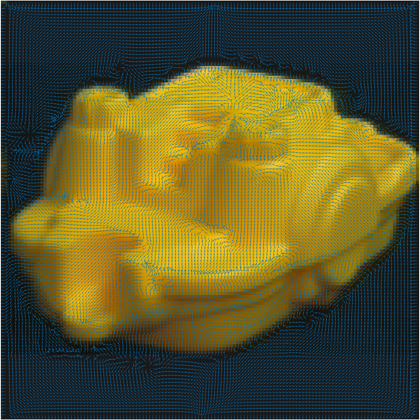
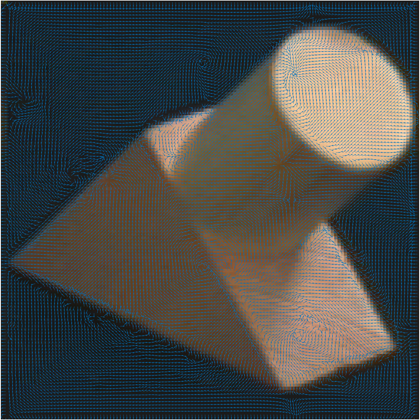
Acknowledgments
We would like to thank the following individuals for their continuing support of this work:
- Department Chair Dr. Tingxiu Wang in the Department of Mathematics at East Texas A&M University
- Mr. Jeremy Gamez, Chief Information Officer, and Mr. Jeff Faunce, Director, Center for IT Excellence at East Texas A&M University
- The team from the Office of Marketing and Communications at East Texas A&M University.
Supporting Publications
See papers [6, 8, 9] by the contributors that validate that augmenting image features increases machine learning effectiveness.